API MPMS 13.3:2017 pdf free download.Manual of Petroleum Measurement Standards Chapter 13.3 Measurement Uncertainty
4.3 True Quantity Value Many quantity values exist; each is a result of a measurement process, and they may not be equal. The true quantity value is that which is determined from a perfect measurement process. All measurement processes are imperfect; therefore, the true quantity value is always unknown. The measured quantity value is the result of an imperfect (realistic) measurement process. 4.4 Error The error is the difference between a measured quantity value and the true quantity value. The true quantity value is unknown; therefore, the error is unknown.
4.4.1 Types of Errors Measurement error may be viewed as having three components of which two are always present: random error and systematic error. The third type of error, gross error, such as misapplication of a procedure; a mistake in recording or analyzing data; or using results from equipment that is malfunctioning can introduce significant error in a measurement result. Such blunders must be identified and data from such must be eliminated from any statistical analysis.
An example of a blunder would be entering a prover volume of 1 0.83 bbls into a flow computer when the calibrated volume was reported as 1 0.38 bbls. Caution: One way of identifying a blunder is to conduct an “outlier test” which is a mathematical procedure to detect unusual results. See Annex F for more details. Random error comes about through unpredictable variations of components that influence the measurement. Random errors appear as different results of apparently repeated identical measurements. The variations of these components are not controlled because of ignorance or by a conscious choice (e.g. it’s judged to be too expensive). Random error cannot be completely eliminated, but it can be reduced by increasing the number of measurements or by using measurement equipment with greater precision. Systematic error comes about from an inaccuracy of components that influence the measurement. Systematic errors include imperfect calibration of measurement equipment, imperfect observation methods or interference with measurement system by the environment.
An example of a systematic error would be to use a volume of 1 0.02 gal for a test measure as reported on the calibration certificate but the true value being 9.99 gal. This error will have the same effect on all measurements involving this test measure and, once known, can be eliminated. Systematic error cannot be completely eliminated but can be reduced by performing more frequent calibrations or by using more precise calibration equipment. The desired outcome of a measurement is a report of the result of the measurement and its associated reliability or uncertainty.
With the advent of publishing the GUM, the focus on improving measurement shifted from dealing with the types of errors associated with a measurement to determining the impact of each error on the total uncertainty of the measurement. This shift avoided the difficultly of categorizing each identified error and the controversy associated with combining the uncertainties of these two types of errors in favor of considering each measurement error as a random variable that can be characterized by a probability distribution from which a standard uncertainty can be determined. Pre-GUM, the terms random and precision as well as the terms systematic and bias were used interchangeably.
Precision is a qualitative description of random errors of the mean of a set of measurements quantified by a statistic such as standard deviation. A related term, accuracy, is a qualitative judgment of closeness to True Value. Accuracy cannot be quantified because the True Value is unknowable. It is possible to have any of the four combinations of (in)accuracy and (im)precision as implied in Figure 1 below.API MPMS 13.3 pdf download.API MPMS 13.3:2017 pdf free download
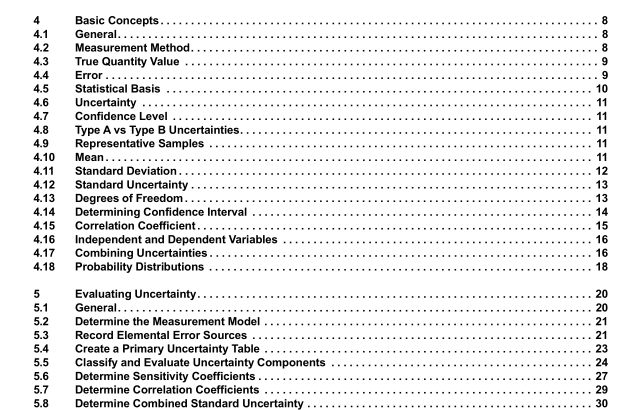